Orthogonal Convolutional Neural Networks
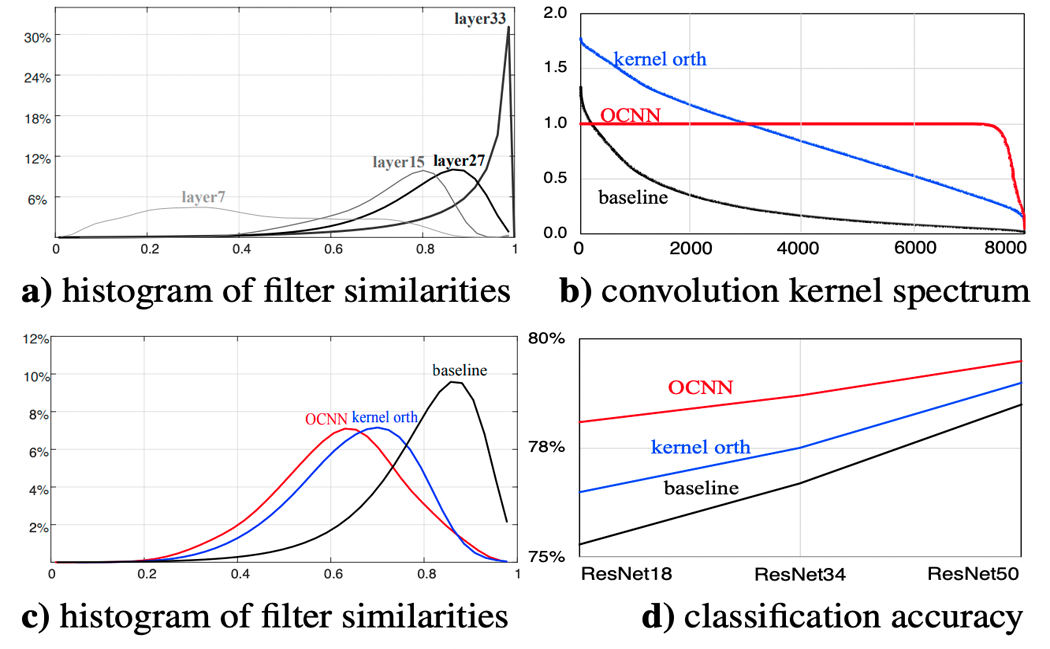
Abstract
Deep convolutional neural networks are hindered by training instability and feature redundancy towards further performance improvement. A promising solution is to impose orthogonality on convolutional filters.
We develop an efficient approach to impose filter orthogonality on a convolutional layer based on the doubly block-Toeplitz matrix representation of the convolutional kernel instead of using the common kernel orthogonality approach, which we show is only necessary but not sufficient for ensuring orthogonal convolutions.
Our proposed orthogonal convolution requires no additional parameters and little computational overhead. This method consistently outperforms the kernel orthogonality alternative on a wide range of tasks such as image classification and inpainting under supervised, semi-supervised and unsupervised settings. Further, it learns more diverse and expressive features with better training stability, robustness, and generalization.
Public Video
Code and Models
Citation
@inproceedings{wang2019orthogonal, title={Orthogonal Convolutional Neural Networks}, author={Wang, Jiayun and Chen, Yubei and Chakraborty, Rudrasis and Yu, Stella X}, booktitle={IEEE Conference on Computer Vision and Pattern Recognition (CVPR)}, year={2020} }